Event
Generative neural network methods for evolutionary inference
Dr. Sara Mathieson
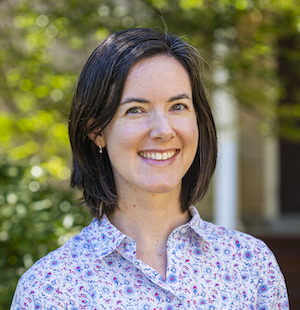
Abstract: Generative AI has captured a worldwide audience with novel text, image, audio, and video. In evolutionary biology we have used synthetic data for decades, for modelling, theoretical development and inference. However, it is often challenging to create realistic simulations for populations with unique histories, which compromises the results of downstream analyses. Recently, new methods inspired by the generative AI literature have emerged as ways to automatically adapt simulations to mirror real genomic data. Here I will focus on our work developing GAN (generative adversarial network) methods that can create simulations matching any population or species. Our fast and flexible algorithms infer demographic parameters such as population sizes and admixture, as well as positive selection and other deviations from neutrality. We apply our methods to data from humans and mosquitoes, identifying novel targets of selection and surprising migration patterns. Many applications of AI are happy to treat models as black boxes, but for scientific purposes we need to understand how they work. This "interpretability" question -- what have complex models learned and what motivates certain predictions -- is the current focus of my lab. I will describe our "model-of-the-model" interpretability approach, as well as discuss next avenues in transformer-based architectures, domain-adaptation and applying machine learning to the population genetics of non-model species.